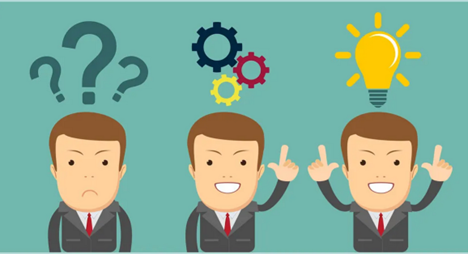
When collaborating with subject matter experts from different business areas, data scientists actively listen for important cues and phrases related to the business problem at hand. They skillfully deconstruct the problem into a well-defined process flow, encompassing a deep comprehension of the underlying business challenge, data requirements and the suitable application of artificial intelligence (AI) and data science techniques for resolution. These fundamental components serve as the building blocks for a series of iterative thought experiments, modeling techniques and assessments aligned with the overarching business objectives.
Throughout the problem-solving journey, it is crucial to maintain a steadfast focus on the business itself. Prematurely introducing technology can potentially divert attention away from the core business problem, resulting in incomplete or misguided solutions.
Achieving success in AI and data science relies heavily on establishing clarity and precision right from the start:
- Clearly articulate and describe the problem that needs to be addressed.
- Precisely define the specific business questions that require answers.
- Identify and incorporate any additional business requirements, such as simultaneously retaining customers while maximizing cross-selling opportunities.
- Quantify the expected benefits in business terms, such as targeting a 10% reduction in churn among high-value customers.
By adhering to these essential practices, data scientists can ensure a purpose-driven approach that is tightly aligned with the business goals, enabling effective problem-solving and delivering meaningful outcomes
The Significance of Well-Defined Problem Statements
In the retail industry, a company sought to understand the factors influencing customer churn. A data science team embarked on the project, aiming to predict customer churn and identify actionable insights to mitigate it. By categorizing customer data, identifying patterns in purchasing behavior and leveraging predictive modeling techniques, they successfully developed a churn prediction model.
This allowed the company to proactively target at-risk customers with personalized retention strategies, resulting in a significant reduction in churn rate and increased customer loyalty. The clear problem statement, focused on predicting customer churn and providing actionable insights, empowered the data scientists to deliver a conclusive and impactful solution.
In the transportation sector, a logistics company wanted to optimize its delivery routes to improve efficiency and reduce costs. Data scientists analyzed historical transportation data, including factors like distance, traffic patterns and package volume. By identifying correlations, clustering delivery regions, and applying optimization algorithms, they developed an optimized routing system. This system enabled the company to streamline its delivery operations, reduce mileage, and enhance customer satisfaction through timely and cost-effective deliveries.
The specific problem statement, centered around route optimization and cost reduction, provided the data scientists with a clear objective to guide their analysis and solution development.
These use case stories highlight how specific and measurable problem statements enable data scientists to apply appropriate techniques and models, leading to actionable insights and tangible outcomes. Whether it’s predicting customer churn, optimizing delivery routes or addressing any other business challenge, a well-defined problem statement is a critical first step towards successful data science solutions.
Type of the problem
Once you’ve identified a problem suitable for data science, it’s essential to determine its type to effectively apply machine learning algorithms. Data science problems generally fall into two categories:
- Supervised Learning: Predicts future outputs using labeled input and output data. Algorithms learn from provided examples to make predictions or classifications on new, unseen data.
- Unsupervised Learning: Uncovers hidden patterns or groupings in unlabeled input data. Algorithms analyze the data to identify underlying structures and relationships without predefined labels or known outcomes.
Understanding the distinction between supervised and unsupervised learning helps data scientists choose the appropriate approach and algorithms for solving their specific problem.
Key Steps in Defining and Framing Data Science Problems
- Identify Key Business Challenges: Start by identifying the critical challenges faced by the organization. These challenges can be related to operational inefficiencies, customer retention, revenue generation, cost reduction, risk management, or any other area where data-driven insights can make a difference.
- Conduct Stakeholder Interviews: Engage with stakeholders from different departments to understand their pain points and requirements. These interviews help gather diverse perspectives and ensure that the problem definition captures the needs of various stakeholders.
- Frame the Problem: Based on the insights gathered, frame the problem statement concisely and clearly. A well-framed problem statement should describe the current state, the desired state, and the specific outcome or insight that the data science project aims to deliver.
- Define Success Metrics: Determine the metrics that will be used to measure the success of the data science solution. Whether it’s increasing conversion rates, reducing customer churn or optimizing operational efficiency, the success metrics should be aligned with the problem statement and organizational goals.
- Set Constraints and Boundaries: Define any constraints or boundaries that may impact the solution. These could include limitations on available data, budget constraints, time limitations, or legal and ethical considerations. Being aware of these constraints upfront helps guide the data science process effectively.
- Validate and Iterate: Share the defined problem statement with stakeholders and seek feedback. Validate that the problem statement accurately captures the business challenge and adjust as necessary. Iteratively refining the problem definition ensures alignment and increases the chances of project success.
Conclusion
Defining business problems is a vital step in the data science journey. It helps organizations focus their efforts, allocate resources wisely, and align data science initiatives with strategic goals. By following a structured approach and involving stakeholders throughout the process, organizations can ensure that their data science projects are targeted, impactful, and ultimately deliver value. Embrace the power of clear problem definition, and you’ll pave the way for effective data-driven solutions that drive business success.